What is AB Testing?
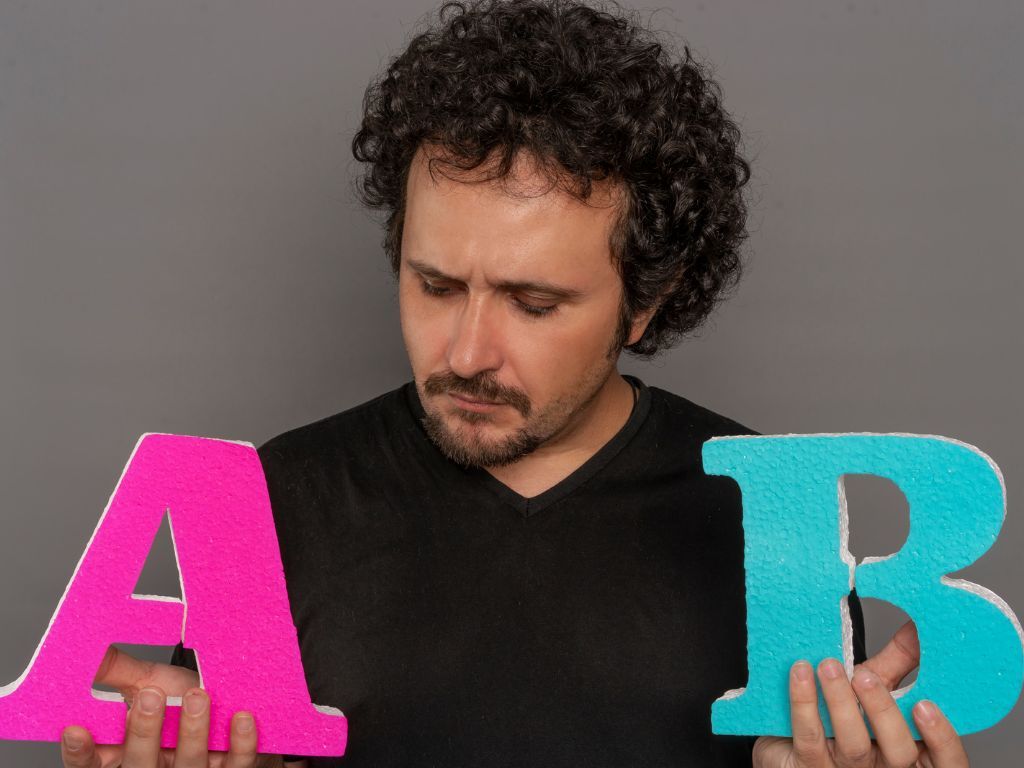
Before I transitioned into the world of data and analytics, I had the opportunity to work in Clinical Operations as a Clinical Trail Associate. It was a demanding role. I was responsible for coordinating and executing clinical trial operations, ensuring the safety of human participants, compliance with regulations, and meeting project goals within budgets and timelines.
For those who don't know, clinical trials are vital to medical research that tests new treatments and interventions and evaluate their effects on human health outcomes. These trials determine the safety and efficacy of new drugs, medical devices, or diets and often compare their effectiveness with existing treatments.
However, despite the importance of the role, the administrative and paperwork-heavy tasks, coupled with extensive travelling, made me realise that it wasn't the right fit for me. The experience taught me that travelling for work vastly differs from travelling for leisure, and the early morning flights and sleep deprivation made it an exhausting experience. I caution those considering travel-heavy roles to think carefully before making a decision.
Moving on to the business side of my company, I thought my clinical knowledge would be irrelevant. But, to my surprise, I came across AB testing, which is very similar to randomised clinical trials. A randomised clinical trial is a technique used to control factors not under direct experimental control by randomly dividing human participants into separate groups. AB testing is a powerful marketing and product development method to test hypotheses and determine the impact of specific interventions or variables on a target population.
In this blog, I would like to share my knowledge of AB testing, specifically on these four points:
- What is AB testing, and how it works?
- Why is AB Testing important, and what are its benefits?
- What are some common use cases for AB testing?
- What are some pitfalls of AB testing
What is AB Testing, and How it Works?
AB testing, also called Split Testing, is a way to determine which version of a webpage or marketing message performs better. Essentially, you show one group of people Version A and another group Version B, and then see which one leads to the most desirable behaviour. This could include clicking a button, purchasing, or filling out a form.
To run an AB test, we first need to divide users or customers into two random groups. Then, each group is shown a different version of the tested element. For example, one group might see a red button while the other sees a green button. We then track the behaviour of each group to see which button leads to more clicks or conversions.
To ensure an accurate result, it's essential to have a large enough sample size and to run the test long enough. Otherwise, we may end up with misleading data.
Why is AB Testing Important, and What are its Benefits?
AB testing is important to businesses as it allows them to optimise their campaigns and product designs for maximum effectiveness. By testing different variables and comparing the results, they can identify which elements resonate with their audience and improve their conversion rates. The benefits of AB testing include increased revenue, better user engagement, and improved customer satisfaction.
Let's take a look at a case study from the book A/B Testing: The Most Powerful way to turn clicks into Customers by Dan Siroker and Pete Koomen. In 2010, the Clinton Bush Haiti Fund was established to raise money for the relief effort following a devastating earthquake in Haiti. The organisation created a donation page to collect donations and conducted AB tests to optimise the page.
The team identified that the website's donation page was the major bottleneck holding back donations. The initial donation page was rather bland, consisting of a long form and many blank spaces on a white background (Figure 1). The team hypothesised that this page was overly abstract. They thought that adding an image of an earthquake victim would make the page more emotive and spur more visitors to donate (Figure 2). However, when they tested this variation against the original, the average donation per page view decreased.
They then hypothesised that the image was pushing the form below the fold, requiring users to scroll, and tried a two-column layout with the image beside the form (Figure 3). This test resulted in significantly more donations than the original and the failing one-column-with-image layout, generating over a million dollars in additional relief aid for Haiti.
As you can see, AB testing allowed the organisation to attract more donations. AB testing has the potential to bring far-reaching benefits to a business.
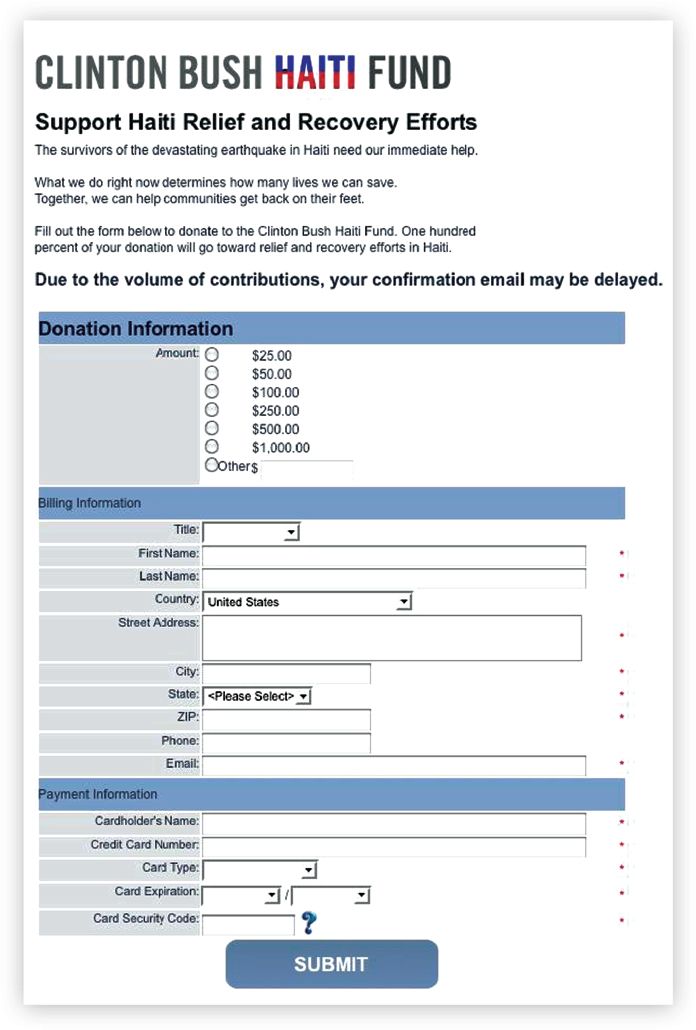
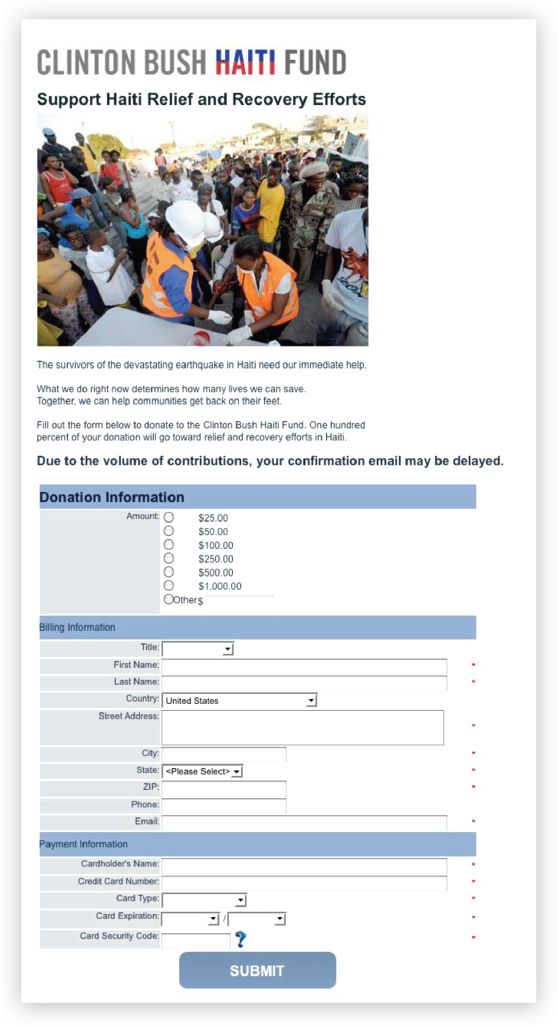
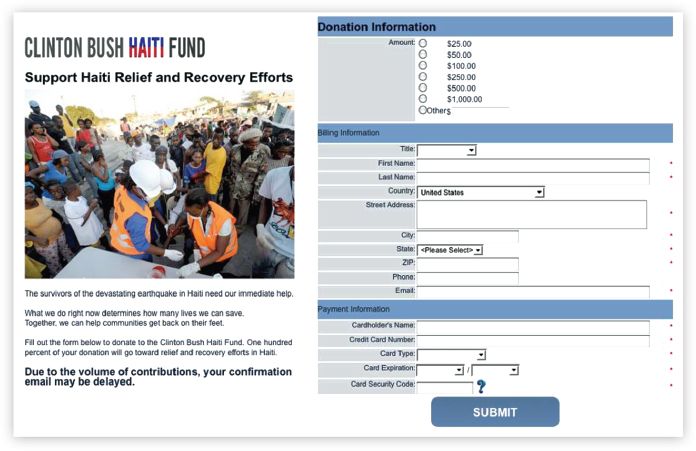
What are Some Common Use Cases for AB Testing?
Here are a few common use cases for AB testing:
-
Website design: A/B Testing can be used to compare two different website designs and determine which is more effective in converting visitors into customers. For example, testing different landing page layouts, colour schemes, or a copy can help identify which design elements resonate best with users.
-
Email campaigns: A/B Testing can also be used to test different email campaign versions to determine which is more likely to result in clicks or conversions. Testing different subject lines, email content, or CTAs can help identify what messaging resonates best with recipients.
-
Pricing: A/B Testing can be used to test different pricing strategies to determine which is more effective in driving sales. For example, testing different price points, discount offers, or payment plans can help identify the optimal pricing strategy.
-
User experience: A/B Testing can be used to test different features or functionality within an app or website to improve user experience. For example, testing different navigation menus or button placements can help identify what design elements result in higher engagement or satisfaction.
-
Advertisements: A/B Testing can be used to test different ad copy, images, or targeting strategies to determine what resonates best with the target audience. This can help optimise ad spend and increase conversions.
What I've listed is not an exhaustive list. A/B Testing can be applied in various scenarios to test variations and measure user behaviour's impact. One of its beauties is that it allows businesses to make data-driven decisions and drive better results.
What are Some of the Common Pitfalls of AB Testing?
While AB testing is awesome, as it can provide valuable insights and help make data-driven decisions, it also has a dark side. AB testing has pitfalls that can lead to inaccurate or misleading results. Here are five of the common pitfalls I've come across in my research:
-
Insufficient sample size: A common mistake in AB testing is using a sample size that is too small to detect meaningful differences between the tested variations. If the sample size is too small, the results may not be statistically significant and may not accurately represent the population.
-
Selection bias: Another common pitfall is selection bias, which occurs when the sample is not representative of the target population. This can happen if the test is conducted on a non-random subset of the population, such as a group of users who are more likely to engage with the website or app.
-
Ignoring external factors: AB testing results can be influenced by external factors, such as changes in the competitive landscape or seasonality. Failing to account for these factors can lead to inaccurate conclusions and suboptimal decisions.
-
Short testing period: A common pitfall is to end the test too early before the variations have had enough time to generate meaningful data. This can lead to false conclusions and suboptimal decisions. Setting an appropriate testing period based on the expected traffic and conversion rates is important.
-
Technical errors: Technical errors can occur during the test setup or data collection, leading to inaccurate results. Examples of technical errors include faulty tracking codes, server errors, or data loss. It is important to monitor the test and ensure the data is collected accurately. Before running AB testing, AA testing can be conducted to test the validity of an AB testing setup. This will help ensure that the testing platform is working correctly and that the results of AB testing are not due to technical issues or biases in the setup.
After reading this blog, I hope you have an understanding of A/B Testing, an appreciation of its values and knowledge of some of the common pitfalls. I know I certainly do, following my research on it. I now need to find an opportunity at work to get my hands dirty and apply this knowledge.